第34届中国数据库学术会议(NDBC2017)IEEE TCDE China Chapter学术沙龙
时间:2017年10月21日 下午13:20-17:35
地址:浙大玉泉校区永谦活动中心主会场
活动介绍
IEEE TCDE携手CCF数据库专委会,依托NDBC平台今后将每年举办一次IEEE TCDE China Chapter学术沙龙活动,邀请当年在IEEE ICDE会议上发表的部分第一单位在中国地区的论文的相关作者来参加活动、并用中文做学术论文报告,希望借此活动加强国内数据库领域顶尖学者之间的交流,并为参与活动的青年教师和学生们提供一个难得的学习机会。
本次活动我们诚意邀请到了IEEE Fellow、国家“千人”计划专家、新南威尔士大学的林学民教授做特邀报告。林教授将在本次特邀报告中不仅将谈及图数据管理等前沿工作,并会站在数据库顶级期刊IEEE TDKE主编的角度给予大家TKDE投稿方面的建议。
此外,当今数据库方向的研究与其他领域交叉结合点甚多,为促进不同领域之间的交流与碰撞,本次活动还邀请到了两位机器学习和自然语言处理方向的专家学者到会报告与交流,希望可以通过不同领域之间的碰撞擦出新的火花。
活动程序委员会
指导委员会主席
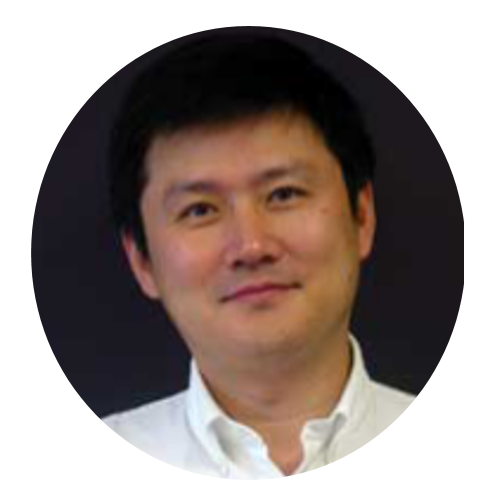
周晓方 IEEE TCDE Chair
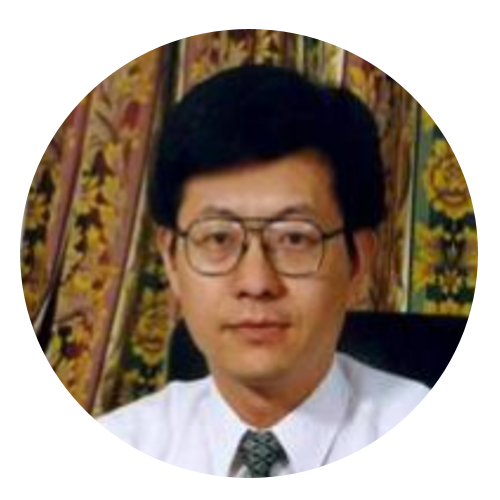
杜小勇 CCF数据库专委会主任
活动主席
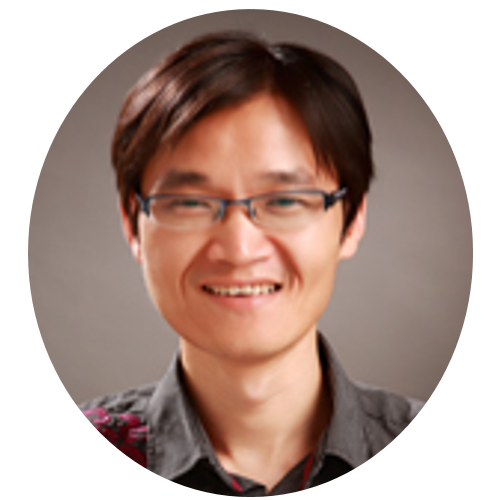
高云君 浙江大学
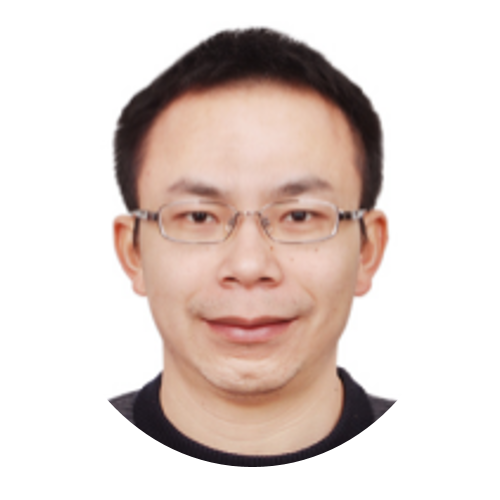
邹磊 北京大学
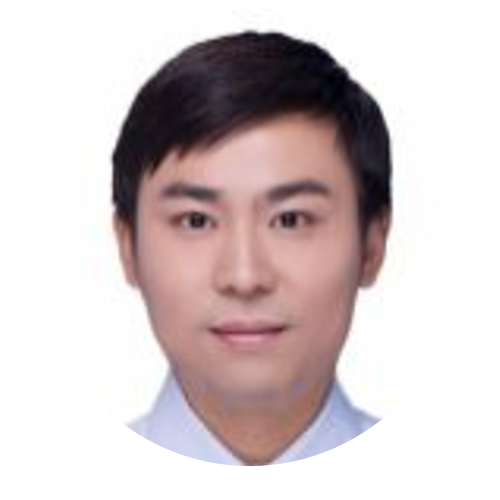
李直旭 苏州大学
日程安排
日期 | 时间 | 主讲人 | 标题 | 单位 |
10月21日 | 13:20-13:30 | 组委会致辞 | ||
13:30-14:20 | 林学民 | 特邀报告 | 新南威尔士大学 | |
14:20-14:40 | 李睿 | Adaptively Secure Conjunctive Query Processing over Encrypted Data for Cloud Computing | 东莞理工学院 & 湖南大学 | |
14:40-15:00 | 成雨蓉 | Complex Event-Participant Planning and Its Incremental Variant | 北京理 工大学 | |
15:00-15:20 | 茶歇 | |||
15:20-16:05 | 沈华伟 | 机器学习领域邀请报告 | 中科院计算所 | |
16:05-16:50 | 冯岩松 | 自然语言处理领域邀 请报告 | 北京大学 | |
16:50-17:10 | 王宁 | PrivSuper: A Superset-First Approach to Frequent Itemset Mining under Differential Privacy | 东北大学 | |
17:10-17:30 | 童咏昕 | Trichromatic Online Matching in Real-time Spatial Crowdsourcing | 北京航空航天大学 | |
17:30-17:35 | 宣布活动结束 |
报名参与方式
本次活动除了特邀讲者和ICDE论文报告作者外,也广泛邀请各位对数据库研究感兴趣的老师、学生和公司在职人员免费注册和参加。具体报名参与方式如下:
请于2017年10月13日前填写好报名表格http://pan.baidu.com/s/1hr9ehTy,并发送至邮箱:tcdechina2017@126.com,邮件主题请写为:注册参加TCDE China活动。
- 重要备注信息:
- 本学术沙龙活动可免费注册参加,食宿请大家自理;
- 本活动得到IEEE TCDE的大力赞助,与会者需免费注册成为IEEE TCDE China会员http://tab.computer.org/tcde/
- 本活动依托NDBC2017举办,建议大家注册并参加NDBC 2017会议;http://www.cs.zju.edu.cn/ndbc2017/registery.html,会议可免费提供餐饮。
- 欢迎大家关注TCDE China微信公众号(报名后即将公布),用于推送TCDE China活动通告。
2017 IEEE TCDE China学术沙龙讲者及报告内容简介
Distinguished Speaker
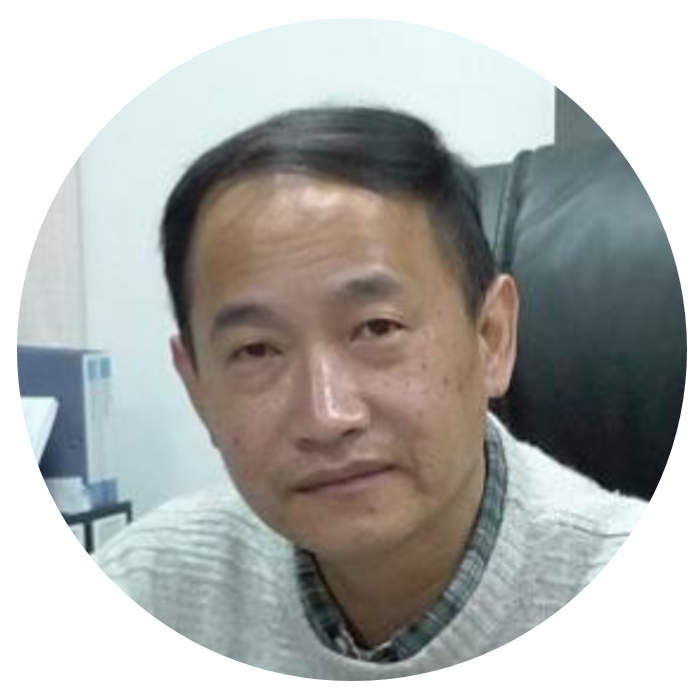
林学民,新南威尔士大学
林学民,新南威尔士大学大学计算机科学及工程学院教授,数据库研究实验室主任,华东师范大学特聘教授。长期从事数据库理论、算法与技术研究,在空间数据、不确定数据、流数据、图数据等领域(和学生及合作者一起)作出了一系列开创性工作,提出了一系列基于海量计算的新颖概念、计算模式及快速有效的索引技术和数据抽取算法。近年来,林学民教授在数据库领域顶级国际学术会议及顶级杂志上共发表了 70 余篇学术论文;累计在数据库和算法领域重要的国际学术会议和国际学术期刊发表并录用了 170 余篇论文,其中 10 篇国际会议优秀论文,包括数据库顶级国际会议 ICDE’07 上的优秀学生论文奖(best student paper award), ICDE’10 上的优秀论文(one of the best papers) , 及数据库顶级国际会议 SIGMOD’11 上的优秀论文(one of the best papers)。他目前的研究兴趣是海量数据的处理,包括图数据、时空数据、字符串数据、不确定数据等。是国际上关于 Skyline 计算、不确定数据等研究方向的先驱者之一。
Invited Talks from External Community
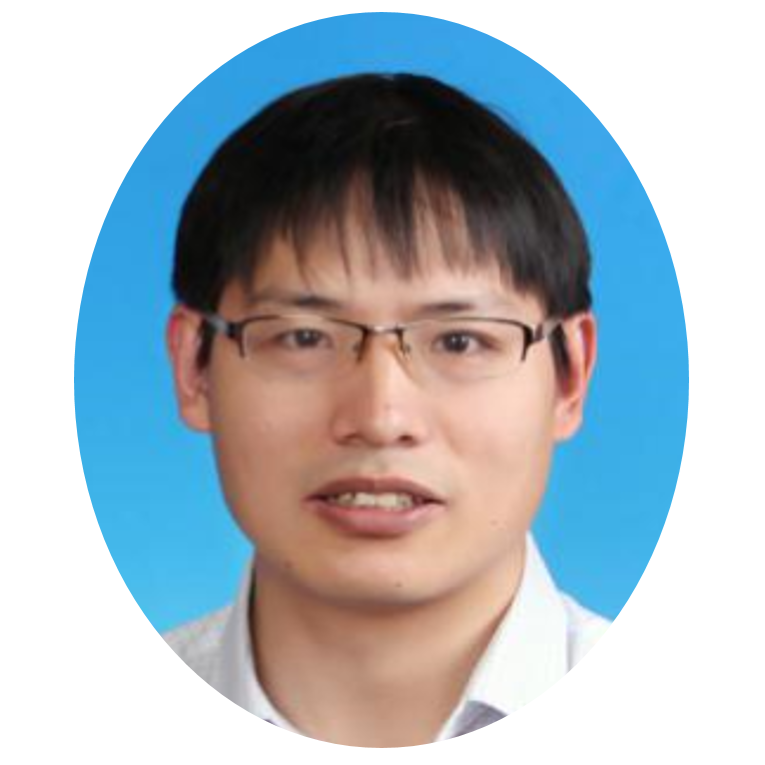
沈华伟,中科院计算所
沈华伟,博士,中国科学院计算技术研究所研究员,中国中文信息学会社会媒体处理专委会副主任。研究方向为网络科学和社会计算。先后获得过CCF优博、中科院优博、首届UCAS-Springer优博、中科院院长特别奖、入选首届中科院青年创新促进会、中科院计算所“学术百星”。2013年在美国东北大学进行学术访问。2015年被评为中国科学院优秀青年促进会会员(中科院优青)。获得国家科技进步二等奖、北京市科学技术二等奖、中国电子学会科学技术一等奖、中国中文信息学会钱伟长中文信息处理科学技术一等奖。出版个人专/译著3部,在网络社区发现、信息传播预测、群体行为分析、学术评价等方面取得了系列研究成果,在Science、PNAS等期刊和WWW、SIGIR、CIKM、WSDM、AAAI、IJCAI等会议上发表论文80余篇,引用2000余次。担任PNAS、IEEE TKDE、ACM TKDD等10余个学术期刊审稿人和WWW、CIKM、WSDM等20余个学术会议的程序委员会委员。
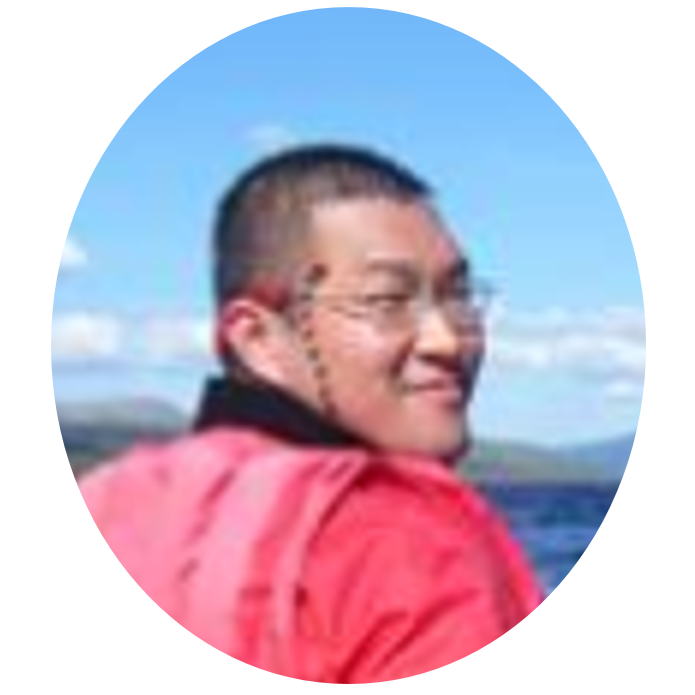
冯岩松,北京大学
冯岩松,博士,北京大学计算机科学与技术学院讲师(助理教授),隶属Web信息处理组。曾在爱丁堡大学与Mirella•Lapata教授合作,期间获得博士学位。在此之前,曾与北京大学的封举富教授合作研究图像识别领域的知识。
ICDE Talks
1)报告题目:Adaptively Secure Conjunctive Query Processing over Encrypted Data for Cloud Computing
讲者简介:李睿,副教授,博士,博士生导师。长期从事数据隐私保护研究,先后在国际顶级会议VLDB、ICDE、INFOCOM、ICDCS、ICNP,国际顶级期刊IEEE/ACM Transaction on Networking, Computer Communication,以及国内一级学报计算机学报、计算机研究与发展、通信学报等会议和杂志上发表科研论文20于篇,取得授权专利一项。主持国家自然科学基金面上项目两项,省部级项目两项。2015年遴选为湖南大学博士生导师,同年遴选为湖南省青年骨干教师。另外,李睿副教授热爱教学工作,先后获得湖南大学教学比赛一等奖、湖南省青年教师教学能手、湖南大学刘銮雄本科教学优秀青年教师奖。指导学生在国际、国家级重要比赛中获奖10于项。
报告简介:This paper concerns the fundamental problem of processing conjunctive queries that contain both keyword conditions and range conditions on public clouds in a privacy preserving manner.No prior Searchable Symmetric Encryption (SSE) based privacy-preserving conjunctive query processing scheme satisfies the three requirements of adaptive security, efficient query processing, and scalable index size. In this paper, we propose the first privacy preserving conjunctive query processing scheme that satisfies the above requirements. To achieve adaptive security, we propose an Indistinguishable Bloom Filter (IBF) data structure for indexing. To achieve efficient query processing and structure indistinguishability, we propose a highly balanced binary tree data structure called Indistinguishable Binary Tree (IBtree). To optimize searching efficiency, we propose a traversal width minimization algorithm and a traversal depth minimization algorithm. To achieve scalable and compact index size, we propose an IBtree space compression algorithm to remove redundant information in IBFs. We formally prove that our scheme is adaptive secure using a random oracle model. The key contribution of this paper is on achieving conjunctive query processing with both strong privacy guarantee and practical efficiency in terms of both speed and space. We implemented our scheme in C++, evaluated and compared its performance with KRB\cite{Seny2013RB} for keyword queries and PBtree\cite{Rui2014PBtree} for range queries on two real-world data sets. Experimental results show that our scheme is fast and scalable (in milliseconds).
2)报告题目:Complex Event-Participant Planning and Its Incremental Variant
讲者简介:Yurong Cheng is a Postdoc in School of Computer Science and Technology at Beijing Institute Technology (BIT), China. She received the BS degree and Ph.D. degree in School of computer science and Engineering from Northeastern University, China. Her main research interests include queries and analysis over graph databases, crowdsourcing, spatio-temporal data processing and analysis, uncertain data management, and approximate query processing.
报告简介:In recent years, online Event Based Social Network (EBSN) platforms have become increasingly popular. One typical task of EBSN platforms is to help users make suitable and personalized plans for participating in different interesting social events. Existing techniques either ignore the minimum participant requirement constraint for each event, which is crucially needed for some events to be held successfully, or assume that events would not change once announced. In this paper, we address the above inadequacies of existing EBSN techniques. We formally define the Global Event Planning with Constraints (GEPC) problem, and its incremental variant. We prove that both are NP-hard, and provide approximate solutions. Finally, we verify the effectiveness and efficiency of our proposed algorithms through extensive experiments over real and synthetic datasets.
3)报告题目:PrivSuper: A Superset-First Approach to Frequent Itemset Mining under Differential Privacy
讲者简介:王宁,东北大学博士研究生。主要研究方向为复杂事件处理、数据清洗和隐私保护。曾参与国家973重点课题和多项国家自然科学基金项目。2014-2015年赴新加坡南洋理工大学联合培养,从事差分隐私技术研究。目前主要致力于移动智能客户端数据收集与隐私保护(Local Differential Privacy)。
报告简介:Differential privacy, which has been applied in Google Chrome and Apple iOS, provides strong privacy assurance to users while retaining the capability to discover statistical patterns from sensitive data. We focus on top-k frequent itemset mining on sensitive data, with the goal of obtaining high result utility while satisfying differential privacy. There are two basic methodologies to design a high-utility solution: one uses generic differential privacy mechanisms as building blocks, and minimizes result error through algorithm design. Most existing work follows this approach. The other methodology is to devise a new building block customized for frequent itemset mining. This is much more challenging: to our knowledge, only one recent work, NoisyCut, attempts to do so; unfortunately, Noisycut has been found to violate differential privacy. We propose a novel solution PrivSuper, which contains both a new algorithm and a new differential privacy mechanism. Unlike most existing methods that follow the Apriori framework, which starts from single items and iteratively forms larger itemsets, PrivSuper directly searches for maximal frequent itemsets, and subsequently adds their sub-itemsets to the results without additional privacy budget consumption. During the search, PrivSuper applies a customized mechanism to extend the current itemset with one more item, which we call the sequence exponential mechanism (SEM). Notably, SEM does not consume any privacy budget at all, if it turns out that the current itemset cannot be extended.
4)报告题目:Trichromatic Online Matching in Real-time Spatial Crowdsourcing
讲者简介:Dr. Yongxin Tong received a Ph.D. degree in Computer Science from the Department of Computer Science and Engineering, The Hong Kong University of Science and Technology (HKUST). He is currently an associate professor in the School of Computer Science and Engineering, Beihang University. Before that, he served as a research assistant professor and a postdoctoral fellow at HKUST. His research interests include crowdsourcing, uncertain data processing and social network analysis. He has published more than 30 papers in highly refereed database and data mining journals and conferences such as SIGMOD, SIGKDD, VLDB, ICDE, TKDE, and TOIS. Dr. Tong was awarded the Microsoft Research Asia Fellowship 2012, and received the Excellent Demonstration Award and the Best Paper Award conferred by the VLDB 2014 and WAIM 2016 conferences, respectively. He has also been a reviewer for leading academic journals, such as VLDBJ, TKDE and TKDD, and has served in the program committees of prestigious international conferences, such as VLDB, ICDE, KDD, AAAI and IJCAI.
报告简介:The prevalence of mobile Internet techniques and Online-To-Offline (O2O) business models has led the emergence of various spatial crowdsourcing (SC) platforms in our daily life. A core issue of SC is to assign real-time tasks to suitable crowd workers. Existing approaches usually focus on the matching of two types of objects, tasks and workers, or assume the static offline scenarios, where the spatio-temporal information of all the tasks and workers is known in advance. Recently, some new emerging O2O applications incur new challenges: SC platforms need to assign three types of objects, tasks, workers and workplaces, and support dynamic real-time online scenarios, where the existing solutions cannot handle. In this paper, based on the aforementioned challenges, we formally define a novel dynamic online task assignment problem, called the trichromatic online matching in real-time spatial crowdsourcing (TOM) problem, which is proven to be NP-hard. Thus, we first devise an efficient greedy online algorithm. However, the greedy algorithm can be trapped into local optimal solutions easily. We then present a threshold-based randomized algorithm that not only guarantees a tighter competitive ratio but also includes an adaptive optimization technique, which can quickly learn the optimal threshold for the randomized algorithm. Finally, we verify the effectiveness and efficiency of the proposed methods through extensive experiments on real and synthetic datasets.