1. 论坛名称
IEEE TCDE China Chapter学术沙龙论坛
2. 论坛组织机构
指导委员会主席:杜小勇(中国人民大学),周晓方(澳大利亚昆士兰大学)
活动主席:邹磊(北京大学),李直旭(苏州大学)
联系人:李直旭(苏州大学)
3. 论坛简介
IEEE TCDE携手CCF数据库专委会,依托NDBC平台将每年举办一次IEEE TCDE China Chapter学术沙龙活动,邀请当年在IEEE ICDE会议上发表的部分第一单位在中国地区的论文的相关作者来参加活动、并用中文做学术论文报告。希望借此活动加强国内数据库领域顶尖学者之间的交流,并为参与活动的青年教师和学生们提供学习与交流的机会。
4. 论坛日程表
5. 论坛特邀报告
特邀报告1:大规模知识图谱构建与应用关键技术
报告摘要:
近年来大数据和人工智能技术飞速发展。以知识图谱为代表的大规模知识库技术在众多应用场景取得显著效果。知识图谱技术发展将知识工程引领进入了大数据时代,知识工程将再次复兴。数据驱动的知识图谱构建与应用技术成为大数据时代知识工程的主要思路。本报告结合复旦大学知识工场实验的一系列研究与落地成果,针对数据驱动的大规模知识库构建,特别是通用百科图谱构建、通用概念图谱构建过程中的知识抽取、质量控制等关键技术展开介绍;针对基于知识图谱的机器语言认知、基于知识图谱搜索与推荐等知识图谱应用关键技术展开介绍。
特邀报告专家简介:
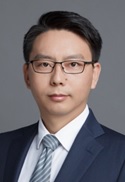
肖仰华博士,复旦大学计算机学院教授、博导、青年973科学家、上海市互联网大数据工程中心执行副主任、上海市数据科学重点实验室知识图谱研究室主任、省部级重点实验室或工程中心专家委员、上市公司等规模企业高级技术顾问或首席科学家。主要研究兴趣包括:大数据管理与挖掘、图数据库、知识图谱等。作为负责人承担30多项各类国家课题与企业课题,至今已经在相关领域顶级、知名国际期刊与会议发表论文70多篇。担任SCI期刊Frontier of Computer Science青年副主编,长期担任众多国际顶级与知名学术会议的程序委员会委员和各类国际知名学术期刊评审人。是ACM、IEEE、AAAI会员和CCF高级会员。领导团队构建国内首个知识库云服务平台(知识工场平台kw.fudan.edu.cn)。该平台目前已经服务相关企业近8亿次API调用。
特邀报告2:智慧教室:多模态大数据在未来课堂的机遇与挑战
报告摘要:
随着科学技术突飞猛进的发展,人们日常生活的各个方面都已经被数字化,各行各业的大数据积累日渐成熟。在教育行业,不论是线下课堂还是线上课堂,整个教学课堂环境都已经被数字化和结构化。传统课堂里的老师和学生的上课行为数据、上课教材的纸质内容等,都被转化成电子版本,结构化的保存了下来,为我们构建智慧教室,打造未来课堂,提供了坚实的基础。与此同时,人工智能技术在无论是在图像、语音还是文本上,都取得了跨时代的突破,让过去遥不可及的梦想变为现实。我们好未来AI实验室,秉承用AI和科技赋能教育的使命,在打造智慧教室的道路上进行了一系列的探索。
此次报告主要分为三个部分:一、教育场景下多模态大数据的收集和回流。这里,我会重点介绍线下数据采集的难点和挑战,以及我们好未来AI实验室是如何打通线下教室数据采集和回流,并分享我们制定的,可复制的良好数据回流规范。二、教育场景下的数据标注和AI建模。这里,我会介绍,如何克服标注数据稀少,标注任务需要专业领域人才等挑战下,如何进行AI建模。同时,我会重点介绍好未来AI实验室分别在计算机视觉、语音情感、自然语言处理、和数据挖掘四个方向上取得的突破性进展和相关成功应用部署的案例。三、建设未来智慧教室面临的机遇和挑战。这里,我会分享一些不鲜为人知的、教育领域独有的数据以及人工智能相关的挑战,希望对大家有指导和启发意义。
特邀报告专家简介:
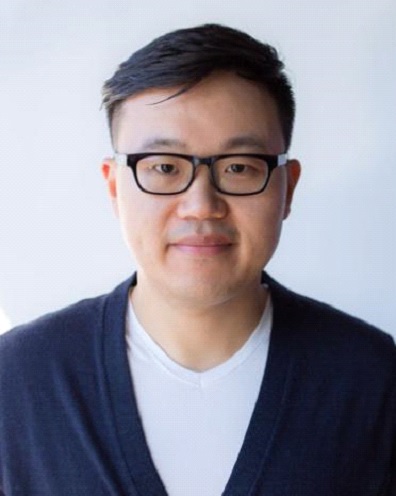
刘子韬,好未来AI实验室机器学习科学家组负责人。美国匹兹堡大学获得计算机专业博士,主要研究方向是机器学习和数据挖掘,在WWW,SIGIR,AAAI,ICDM,ECML等顶级会议发表论文数十篇。2016年加入美国Pinterest公司,任职研究科学家,主要负责Pinterest的图片推荐和广告竞价等业务。之前曾供职于Google, eBay Research Lab,Yahoo!Lab,Alibaba iDST。
6. ICDE 2018优秀论文报告
1)报告题目:A GPU-accelerated Framework for Processing Trajectory Queries
讲者简介:沈艳艳,女,上海交通大学计算机科学及工程系副研究员。2010年8月新加坡国立大学博士毕业。主要研究领域包含数据库技术、海量数据分析、数据驱动的机器学习等。在SIGMOD、VLDB、ICDE、AAAI、IJCAI等国际顶级会议和期刊发表论文三十余篇。担任过VLDB、ICDE、IJCAI等重要国际会议的程序委员会委员。主持国家自然科学基金青年项目1项,作为骨干成员参与领域相关的863项目1项。
报告简介:The increasing amount of trajectory data facilitates a wide spectrum of practical applications. In many such applications, large numbers of trajectory range and similarity queries are issued continuously, which calls for high-throughput trajectory query processing. Traditional in-memory databases lack considerations of the unique features of trajectories, thus suffering from inferior performance. Existing trajectory query processing systems are typically designed for only one type of trajectory queries, i.e., either range or similarity query, but not for both. Inspired by the massive parallelism on GPUs, in this paper, we develop a GPU-accelerated framework, named GAT, to support both types of trajectory queries (i.e., both range and similarity queries) with high throughput. For similarity queries, we adopt the Edit Distance on Real sequence (EDR) as the similarity measure which is accurate and robust to noise in realworld trajectories. GAT employs a GPU-friendly index called GTIDX to effectively filter invalid trajectories for both range and similarity queries, and exploits the GPU to perform parallel verifications. To accelerate the verification process on the GPU, we apply the Morton-based encoding method to reorganize trajectory points and facilitate coalesced data accesses for individual point data in global memory, which reduces the global memory bandwidth requirement significantly. We also propose a technique of grouping size-varying cells into balanced blocks with similar numbers of trajectory points, to achieve load balancing among the Streaming Multiprocessors (SMs) of the GPU. We conduct extensive experiments to evaluate the performance of GAT using two real-life trajectory datasets. The results show that GAT is scalable and achieves high throughput with acceptable indexing cost.
2)报告题目:Range Optimistic Concurrency Control for a Composite OLTP and Bulk Processing Workload
讲者简介:蔡鹏,2011年在华东师范大学获得博士学位。2015年6月加入华东师范大学,在此之前先后就职于IBM中国研究院和百度(中国)有限公司。研究兴趣包括高性能内存事务处理,高可用机制,基于机器学习/统计模型的自我管理数据库系统。
报告简介:This work addresses the need for efficient key-range validation for a composite OLTP and bulk processing workload characterized by modern enterprise applications. In-memory database system (IMDB), mostly adopting the optimistic concurrency control (OCC) mechanism, performs well if the contention of conventional OLTP workloads is low and each transaction only contains point read/write query with primary key. In this work we present the performance problem of IMDBs under mixed OLTP and bulk processing workloads. The reason is that existing OCC protocols take expensive cost to generate a serializable schedule if the OLTP workload contains bulk processing operations with key-range scan.
To this end, we develop an efficient and scalable range optimistic concurrency control (ROCC) which uses logical ranges to track the potential conflicting transactions and to reduce the number of transactions to be validated.
At the read phase, a transaction keeps a set of predicates to remember the version and precise scope of scanned ranges, which eliminates the cost of maintaining scanned records. Before entering the validation phase, if the transaction intends to update records in the logical range, it needs to register to the corresponding lock-free list implemented by a circular array. Finally, ROCC filters out unrelated transactions and validates the bulk operation at range level.
Experimental results show that ROCC has good performance and scalability under heterogeneous workloads mixed with point access and bulk processing.
3)报告题目:Rule-Based Graph Repairing: Semantic and Efficient Repairing Methods
讲者简介:Yurong Cheng received the BS and PhD degree in College of Computer Science and Engineering of Northeastern University, China, in 2012 and 2017 respectively. Currently, she is a Postdoc in Computer Science of Beijing Institute of Technology. Her main research interests include queries and analysis over uncertain graphs, knowledge bases, social networks, and spatiotemporal databases.
报告简介:Real-life graph datasets extracted from Web are inevitably full of incompleteness, conflicts, and redundancies, so graph data cleaning shows its necessity. One of the main issues is to automatically repair the graph with some repairing rules. Although rules like data dependencies have been widely studied in relational data repairing, very few works exist to repair the graph data. In this paper, we introduce an automatic repairing semantic for graphs, called Graph-Repairing Rules (GRRs). This semantic can capture the incompleteness, conflicts, and redundancies in the graphs and indicate how to correct these errors. We study three fundamental problems associated with GRRs, implication, consistency and termination, which show whether a given set of GRRs make sense. Repairing the graph data using GRRs involves a problem of finding isomorphic subgraphs of the graph data for each GRR, which is NP-complete. To efficiently circumvent the complex calculation of subgraph isomorphism, we design a decomposition-and-join strategy to solve this problem. Extensive experiments on real datasets show that our GRR semantic and corresponding repairing algorithms can effectively and efficiently repair real-life graph data.
4)报告题目:Finding Top-k Optimal Sequenced Routes
讲者简介:金澈清,华东师范大学数据科学与工程学院副院长,教授,博士生导师,中国计算机学会数据库专委会委员。研究兴趣主要包括数据流管理、基于位置的服务、不确定数据管理、区块链技术等。主持多项国家自然科学基金;已出版英文专著1部、参与翻译《海量数据分析前沿》和《Hadoop权威指南》(第2版和第3版);发表论文80余篇,其中多篇论文获得优秀论文奖励,包括《计算机学报》优秀论文奖、WAIM 2011最佳论文提名奖等。曾获得霍英东教育基金会青年教师奖。
报告简介:Motivated by many practical applications in logistics and mobility-as-a-service, we study the top-k optimal sequenced routes (KOSR) querying on large, general graphs where the edge weights may not satisfy the triangle inequality, e.g., road network graphs with travel times as edge weights. The KOSR querying strives to find the top-k optimal routes (i.e., with the top-k minimal total costs) from a given source to a given destination, which must visit a number of vertices with specific vertex categories (e.g., gas stations, restaurants, and shopping malls) in a particular order (e.g., visiting gas stations before restaurants and then shopping malls).
To efficiently find the top-k optimal sequenced routes, we propose two algorithms PruningKOSR and StarKOSR. In PruningKOSR, we define a dominance relationship between two partially-explored routes. The partially-explored routes that can be dominated by other partially-explored routes are postponed being extended, which leads to a smaller searching space and thus improves efficiency. In StarKOSR, we further improve the efficiency by extending routes in an A∗ manner. With the help of a judiciously designed heuristic estimation that works for general graphs, the cost of partially explored routes to the destination can be estimated such that the qualified complete routes can be found early. In addition, we demonstrate the high extensibility of the proposed algorithms by incorporating Hop Labeling, an effective label indexing technique for shortest path queries, to further improve efficiency. Extensive experiments on multiple real-world graphs demonstrate that the proposed methods significantly outperform the baseline method. Furthermore, when k = 1, StarKOSR also outperforms the state-of-the-art method for the optimal sequenced route queries.
5)报告题目:Efficient Signed Clique Search in Signed Networks
讲者简介:李荣华博士于2013年8月毕业于香港中文大学,同年9月加入深圳大学计算机与软件学院,2018年3月调入北京理工大学计算机学院工作。李博士的主要研究兴趣包括图数据挖掘、社交网络分析与挖掘、基于图的机器学习、图数据库、以及图计算系统等。在上述相关领域,李博士发表学术论文30余篇,其中大多数论文发表在数据库和数据挖掘领域的著名会议SIGMOD、VLDB、KDD、ICDE,以及著名期刊VLDB Journal、TKDE上。李博士担任了著名会议KDD、WWW、ICDE、SDM、CIKM等的程序委员会委员,长期担任ACM TODS、VLDB Journal、 IEEE TKDE、ACM TKDD等著名期刊的审稿人。李博士是中国计算机学会数据库专业委员会的委员。
报告简介:Mining cohesive subgraphs from a network is a fundamental problem in network analysis. Most existing cohesive subgraph models are mainly tailored to unsigned networks. In this paper, we study the problem of seeking cohesive subgraphs in a signed network, in which each edge can be positive or negative, denoting friendship or conflict respectively. We propose a novel model, called maximal (α, k)-clique, that represents a cohesive subgraph in signed networks. Specifically, a maximal (α, k)-clique is a clique in which every node has at most k negative neighbors and at least αk positive neighbors (α≥1). We show that the problem of enumerating all maximal (α, k)-cliques in a signed network is NP-hard. To enumerate all maximal (α, k)-cliques efficiently, we first develop an elegant signed network reduction technique to significantly prune the signed network. Then, we present an efficient branch and bound enumeration algorithm with several carefully-designed pruning rules to enumerate all maximal (α, k)-cliques in the reduced signed network. The results of extensive experiments on five large real-life datasets demonstrate the efficiency, scalability, and effectiveness of our algorithms.
6)报告题目:Efficient Learning Interpretable Shapelets for Accurate Time Series Classification
讲者简介:王鹏,复旦大学计算机科学技术学院副教授,博导。主要研究兴趣包括:大数据管理与挖掘、物联网大数据管理、智能运维数据分析等。973青年科学家。2012年获得教育部自然科学二等奖(第三完成人)。主持或主要参与科技部重点研发专项、国家青年973、自然科学重点/面上基金、上海市科委、上海市经信委的多个项目,以及IBM、EMC、爱立信、华为等企业的资助项目。在数据库领域顶级国际期刊与会议SIGMOD、VLDB、ICDE、TKDE、ICDM等发表论文20多篇。曾担任众多国际顶级与知名学术会议的程序委员会委员,包括SIGKDD、ICDE、DASFAA、WAIM等。国家自然科学基金的评审专家。国际知名学术期刊TKDE、KAIS、JCST等的评审人。
报告简介:Recently, time series classification with shapelets, due to their high discriminative ability and good interpretability, has attracted considerable interests within the research community. Previously, shapelet generating approaches extracted shapelets from training time series or learned shapelets with many parameters. Although they can achieve higher accuracy than other approaches, they still confront some challenges. First, searching or learning shapelets in the raw time series space incurs a huge computation cost. For example, it may cost several hours to deal with only hundreds of time series. Second, they must determine how many shapelets are needed beforehand, which is difficult without prior knowledge. To overcome these challenges, in this paper, we propose a novel algorithm to learn shapelets. We first discover shapelet candidates from the Piecewise Aggregate Approximation (PAA) word space, which is much more efficient than searching in the raw time series space. Moreover, the concept of coverage is proposed to measure the quality of candidates, based on which we design a method to compute the optimal number of shapelets. After that, we apply the logistic regression classifier to adjust the shapelets. Extensive experimentation on 15 datasets demonstrates that our algorithm is more accurate against 6 baselines and outperforms 2 orders of magnitude in terms of efficiency. Moreover, our algorithm has fewer redundant shape-like shapelets and is more convenient to interpret classification decisions.
7)报告题目:Enabling Quality Control for Entity Resolution: A Human and Machine Cooperation Framework
讲者简介:陈群,男,1976年出生,清华大学管理信息系统学士,新加坡国立大学计算机科学博士,现任西北工业大学教授,博士生导师。研究领域为大数据与人工智能。曾获得国家教育部“新世纪优秀人才”和劳动与社会保障部“海外高层次引进人才”称号。计算机学会数据库专家委员会委员,国家自然科学基金委信息学科数据库与大数据领域评审专家,科技部重点研发专项大数据与人工智能领域专家。曾经或正在主持自然科学基金委青年基金项目, 重点项目和国际合作项目;科技部863和重点研发课题等8个国家级课题。在国内外专业权威期刊发表近80篇学术文章,其中多篇文章发表在代表领域最高水平的顶级会议或期刊SIGMOD, ICDE, WWW, CIKM, ICDM, ICDCS和TKDE等。
报告简介:Even though many machine algorithms have been proposed for entity resolution, it remains very challenging to find a solution with quality guarantees. In this paper, we propose a novel HUman and Machine cOoperation (HUMO) framework for entity resolution (ER), which divides an ER workload between the machine and the human. HUMO enables a mechanism for quality control that can flexibly enforce both precision and recall levels. We introduce the optimization problem of HUMO, minimizing human cost given a quality requirement, and then present three optimization approaches: a conservative baseline one purely based on the monotonicity assumption of precision, a more aggressive one based on sampling and a hybrid one that can take advantage of the strengths of both previous approaches. Finally, we demonstrate by extensive experiments on real and synthetic datasets that HUMO can achieve high-quality results with reasonable return on investment (ROI) in terms of human cost, and it performs considerably better than the state-of-the-art alternatives in quality control.
8)报告题目:Continuous Proximity Detection via Predictive Safe Region
讲者简介:张东祥博士毕业于新加坡国立大学,现为电子科技大学“校百人”教授,主要从事时空大数据分析、智慧城市、智能教育等前沿课题的相关研究,目前担任中国计算机学会数据库专业委员会委员,2016年入选四川省“千人计划”青年人才项目。已发表50余篇高水平论文,其中40篇为CCF A类论文,第一/通讯作者18篇,Google Scholar引用超过1100次,第一作者的论文单篇最高引用超过300次,H指数17,国家发明专利受理6项。多次担任重要数据库会议的程序委员会委员(包括ICDE 2012,2018、APWeb 2015-2016、WAIM 2014-2016、SSTD 2015和NDBC 2016-2018),并长期在IEEE Transactions on Knowledge and Data Engineering、ACM Transactions on Information Systems和VLDB Journal等CCF A类期刊担任审稿人员。论文《Effective Multi-Modal Retrieval based on Stacked Auto-Encoders》获VLDB 2014年度最佳论文候选;论文《CANDS: Continuous Optimal Navigation via Distributed Stream Processing》获上海市CCF数据库与数据挖掘2015年度候选优秀论文。
报告简介:Continuous proximity detection monitors the real-time positions of a large set of moving users and sends an alert as long as the distance of any matching pair is smaller than the threshold. Existing solutions construct either a static safe region with maximized area or a mobile safe region with constant speed and direction, which cannot not capture real motion patterns. In this paper, we propose a new type of safe region that relies on trajectory prediction techniques to significantly reduce the communication I/O. It takes into account the complex non-linear motion patterns and constructs a stripe to enclose the sequence of future locations as a predictive safe region. The stripe construction is guided by a holistic cost model with the objective of maximizing the expected time for the next communication. We conduct experiments on four real datasets with four types of prediction models and our method reduces the communication I/O by more than 30% in the default parameter settings.
9)报告题目:Diversified Coherent Core Search on Multi-Layer Graphs
讲者简介:Rong Zhu is a soon-to-graduate PhD student in Harbin Institute of Technology under the supervision of Prof. Jianzhong Li and Prof. Zhaonian Zou. His main research interests include graph databases, graph data mining and social network analysis. He has published nearly 10 papers in referred journals and conferences such as TKDE, KAIS,ICDE and ICDM. He has been awarded the IBM PhD Fellowship and nominated for the Microsoft Research Asia Fellowship in 2016, and received the Baidu Scholarship in 2018.
报告简介:Mining dense subgraphs on multi-layer graphs is an interesting problem, which has witnessed lots of applications in practice. To overcome the limitations of the quasi-clique-based approach, we propose d-coherent core (d-CC), a new notion of dense subgraph on multi-layer graphs, which has several elegant properties. We formalize the diversified coherent core search (DCCS) problem, which finds k d-CCs that can cover the largest number of vertices. We propose a greedy algorithm with an approximation ratio of 1 - 1/e and two search algorithms with an approximation ratio of 1/4. The experiments verify that the search algorithms are faster than the greedy algorithm and produce comparably good results as the greedy algorithm in practice. As opposed to the quasi-clique-based approach, our DCCS algorithms can fast detect larger dense subgraphs that cover most of the quasi-clique-based results.