Conference
- Home
- Keynotes
- Tutorials
- Workshops
- Data Challenge
- Accepted Papers
- Conference Program
- Conference Venue
- Student Travel Award
Author Information
- Call for Contributions
- Submission Guideline
- Important Dates
- Registration
- Accommodation
- Transportation
Organization
Sponsors
Keynotes
Keynote Session 1: Big Data - Security and Privacy
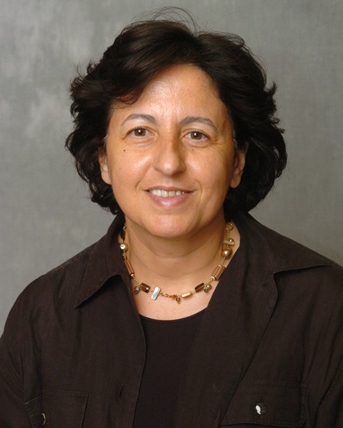
Elisa Bertino: professor at the Department of Computer Science, Purdue University, Director of the Cyber Center (Discovery Park) and Research Director of CERIAS.
Abstract: Technological advances and novel applications, such as sensors, cyber-physical systems, smart mobile devices, cloud systems, data analytics, and social networks, are making possible to capture, and to quickly process and analyze huge amounts of data from which to extract information critical for security-related tasks. In the area of cyber security, such tasks include user authentication, access control, anomaly detection, user monitoring, and protection from insider threat. By analyzing and integrating data collected on the Internet and Web one can identify connections and relationships among individuals that may in turn help with homeland protection. By collecting and mining data concerning user travels and disease outbreaks one can predict disease spreading across geographical areas. And those are just a few examples; there are certainly many other domains where data technologies can play a major role in enhancing security. The use of data for security tasks is however raising major privacy concerns. Collected data, even if anonymized by removing identifiers such as names or social security numbers, when linked with other data may lead to re-identify the individuals to which specific data items are related to. Also, as organizations, such as governmental agencies, often need to collaborate on security tasks, data sets are exchanged across different organizations, resulting in these data sets being available to many different parties. Apart from the use of data for analytics, security tasks such as authentication and access control may require detailed information about users. An example is multi-factor authentication that may require, in addition to a password or a certificate, user biometrics. Recently proposed continuous authentication techniques extend access control system. This information if misused or stolen can lead to privacy breaches. It would then seem that in order to achieve security we must give up privacy. However this may not be necessarily the case. Recent advances in cryptography are making possible to work on encrypted data-for example for performing analytics on encrypted data. However much more needs to be done as the specific data privacy techniques to use heavily depend on the specific use of data and the security tasks at hand. Also, current techniques are not still able to meet the efficiency requirement for use with big data sets. In this talk we will discuss methods and techniques to make this reconciliation possible and identify research directions.
Biography: Elisa Bertino is professor of computer science at Purdue University, and serves as Director of Purdue Cyber Center and Research Director of the Center for Information and Research in Information Assurance and Security (CERIAS). She is also an adjunct professor of Computer Science & Info tech at RMIT. Prior to joining Purdue in 2004, she was a professor and department head at the Department of Computer Science and Communication of the University of Milan. She has been a visiting researcher at the IBM Research Laboratory (now Almaden) in San Jose, at the Microelectronics and Computer Technology Corporation, at Rutgers University, at Telcordia Technologies. Her recent research focuses on database security, digital identity management, policy systems, and security for web services. She is a Fellow of ACM and of IEEE. She received the IEEE Computer Society 2002 Technical Achievement Award and the IEEE Computer Society 2005 Kanai Award. She is currently serving as EiC of IEEE Transactions on Dependable and Secure Computing
Keynote Session 2: Personalized Web News Filtering and Summarization
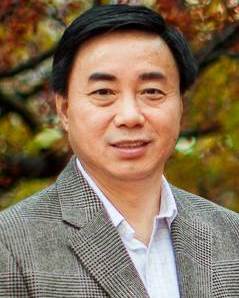
Xindong Wu: Professor, Department of Computer Science, University of Vermont, USA.
Abstract: Information on the World Wide Web is congested with large amounts of news contents. Recommending, filtering, and summarization of Web news have become hot topics of research in Web intelligence, aiming to find interesting news for users and give concise content for reading. This talk presents our research on developing the Personalized News Filtering and Summarization system (PNFS). An embedded learning component of PNFS induces a user interest model and recommends personalized news. Two Web news recommendation methods are proposed to keep tracking news and find topic interesting news for users. A keyword knowledge base is maintained and provides real-time updates to reflect the news topic information and the users interest preferences. The non-news content irrelevant to the news Web page is filtered out. A keyword extraction method based on lexical chains is proposed that uses the semantic similarity and the relatedness degree to represent the semantic relations between words. Word sense disambiguation is also performed in the built lexical chains. Experiments on Web news pages and journal articles show that the proposed keyword extraction method is effective. An example run of our PNFS system demonstrates the superiority of this Web intelligence system.
Biography: Xindong Wu is a Yangtze River Scholar in the School of Computer Science and Information Engineering at the Hefei University of Technology (China), a Professor of Computer Science at the University of Vermont (USA), and a Fellow of the IEEE and AAAS. He received his Bachelor's and Master's degrees in Computer Science from the Hefei University of Technology, China, and his Ph.D. degree in Artificial Intelligence from the University of Edinburgh, Britain. His research interests include data mining, knowledge-based systems, and Web information exploration. Dr. Wu is the Steering Committee Chair of the IEEE International Conference on Data Mining (ICDM), the Editor-in-Chief of Knowledge and Information Systems (KAIS, by Springer), and an Editor-in-Chief of the Springer Book Series on Advanced Information and Knowledge Processing (AI&KP). He was the Editor-in-Chief of the IEEE Transactions on Knowledge and Data Engineering (TKDE, by the IEEE Computer Society) between 2005 and 2008. He served as Program Committee Chair/Co-Chair for ICDM '03 (the 2003 IEEE International Conference on Data Mining), KDD-07 (the 13th ACM SIGKDD International Conference on Knowledge Discovery and Data Mining), CIKM 2010 (the 19th ACM Conference on Information and Knowledge Management), and IEEE/ACM ASONAM '14 (the 2014 IEEE/ACM International Conference on Advances in Social Network Analysis and Mining). He received the 2012 IEEE Computer Society Technical Achievement Award "for pioneering contributions to data mining and applications", and the 2014 IEEE ICDM 10-Year Highest-Impact Paper Award.
Keynote Session 3: Querying Big Data: From Theory and Practice
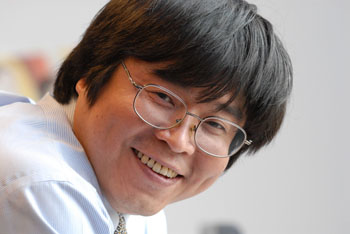
Wenfei Fan: Professor of Web Data Management, LFCS, School of Informatics, University of Edinburgh, UK.
Abstract: Querying big data is a departure from our familiar database techniques and even from the classical computational complexity theory. Several questions arise. What query classes are tractable in the context of big data? When is it feasible to query big data by accessing small data? Is there any practical technique for querying big data? When exact answers are beyond reach in practice, is approximate query answering possible within our constrained resources and with accuracy guarantees? This talk aims to provide an overview of recent advances in tackling these questions.
Biography: Professor Wenfei Fan is the Chair of Web Data Management in the School of Informatics, University of Edinburgh, UK, and the director of the International Research Center on Big Data, Beihang University, China. Prior to his move to the UK, he worked for Bell Labs, Lucent Technologies in the USA. He received his PhD from the University of Pennsylvania, USA, and his MS and BS from Peking University, China. Professor Fan is a Fellow of the Royal Society of Edinburgh, UK, a Fellow of the ACM, USA, a National Professor of the 1000-Talent Program and a Yangtze River Scholar, China. He is a recipient of the Alberto O. Mendelzon Test-of-Time Award of ACM PODS 2015 and 2010, ERC Advanced Fellowship in 2015, the Roger Needham Award in 2008, the Outstanding Overseas Young Scholar Award in 2003, the NSF Career Award in 2001, and several Best Paper Awards (VLDB 2010, ICDE 2007, and Computer Networks 2002). His current research interests include database theory and systems, in particular big data, data quality, data integration, distributed query processing, query languages, recommender systems, social networks and Web services.